Three Things to Consider for Responsible AI in Government
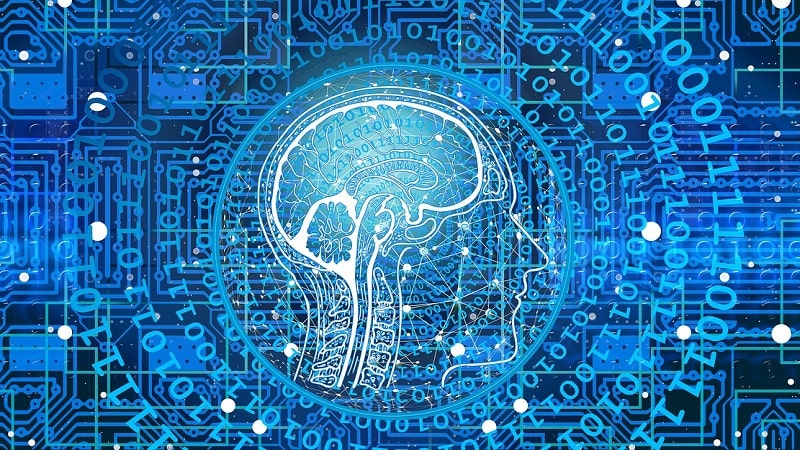
The use of AI and analytics are crucial for government agencies, who are among the largest owners of data. The benefits of AI are often discussed – operational efficiencies, intelligent automation possibilities and the ability to gain deeper insights from massive amounts of data.
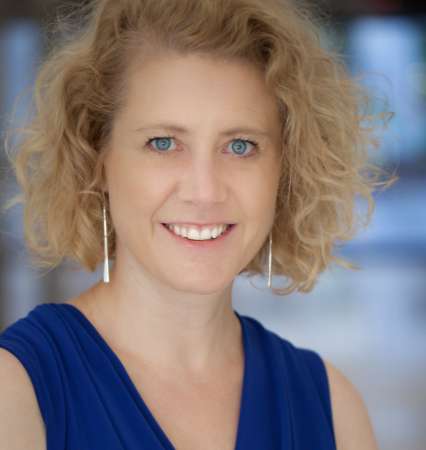
With the intense interest and proliferation of AI, governance of machine intelligence is getting more attention and appropriately so. Absent legislation, organizations must anticipate and adopt voluntary practices to minimize risk and avoid undesirable outcomes.
Here are three areas of focus recommended as part of a comprehensive responsible AI strategy.
Plan Ahead
Responsible AI solutions start with planning. Some key questions to ask (and then answer) during the initiation of an AI project are:
- What is the intended use case and are there other unintended uses that may need to be mitigated against?
- What are the expected outcomes of the AI solution and are there possible unintentional impacts on individuals or community welfare? Are these positive or negative impacts?
- How is the data used in the AI solution monitored and managed? Are data governance policies defined and applied consistently? Is data quality consistent and at appropriate level of completeness?
- Where is there potential for bias with the AI solution and how can this be monitored and managed?
- What considerations are needed to create model transparency and explainability?
Similar to applying the DevSecOps mindset, where teams “shift left” on security planning and execution to include these activities from the start, the same is recommended for risk planning in AI projects. Identify potential challenges and risks early and commit to maintaining a plan to assess and address them.
Be Transparent
AI models are complex, and transparency into how machine intelligence is making decisions and taking action is becoming increasingly critical. AI models now help us drive more safely through real-time alerts, or in some cases drive for us. AI is being incorporated into medical research and treatment plans. This level of complexity can be difficult to decipher when systems don’t operate with expected outcomes. What went wrong? Why was a decision made or an action taken?
Explainable AI (or XAI) advocates for fully transparent AI solutions. This means that all code and workflows can be interpreted and understood without having advanced technical knowledge. This often requires additional steps in the design and build of the solution to ensure explainability is achieved and maintained.
Think of explainability as a two-step process – first, interpretability, the ability to interpret an AI model, and second, explainability, to be able to explain it in a way humans can comprehend. Explainable models provide transparency – so organizations stay accountable to users or customers and build trust over time. A black box solution that cannot be interpreted when things go awry is high risk investment that is potentially damaging and unexpectedly more expensive.
Enable Humans in the Loop
The Toyota production line Andon Cord is famous for its ability to stop the production line in the pursuit of quality. A physical rope used to halt all work when a defect was suspected, enabling an assessment and resolution of the issue before it can proliferate further.
What is the equivalent in the build and use of possibly high-stakes automated AI solutions? A human in the loop – enabling the ability for a person to oversee and have the option to override the system outputs. This can include data labeling by humans to support the model training process, human involvement in validating model results to support model “learning,” and implementing monitoring and alerts that require human review when specific or unexpected conditions are detected.
The combination of human and machine intelligence is a powerful one that expands possibilities while enacting safeguards.
By implementing governance guidelines and adopting approaches that specifically address the challenges and risks associated with AI solutions, federal organizations can proactively act to protect the interests of the public and Federal employees.