The chief data officer of the U.S. Air Force’s SEEK EAGLE Office (AFSEO) – which certifies weapons and other aircraft-attached equipment using digital modeling, simulation and analysis, and ground and flight tests – reported last week on progress that the organization is making in overhauling data management practices with the benefit of machine learning and other technologies.
Speaking at an August 21 Massachusetts Institute of Technology event, AFSEO CDO Donna Cotton discussed the 33-year old organization’s work in testing how aircraft perform with various attached payloads. With much of that work, data generated by engineers has been siloed according to projects, has been difficult to locate, and has not been easily re-used across projects.
Cotton’s job is to provide architecture development, data management, and cybersecurity for AFSEO, and she leads data transformation efforts including advanced analytics and artificial intelligence/machine learning capabilities to manage over 100 million files of critical aircraft store compatibility data.
“We rely on our old data very heavily,” she said. “The big win for AFSEO is the ability to connect all that data together,” Cotton said, adding that the net result of better data management is “going from eight teams working independently to one team producing a product,” and providing for use of data across disciplines within the organization.
Citing the Air Force’s larger goal of leveraging engineering data as a strategic asset, she said data digitization and data accessibility are central to reaching that goal. For example, she said, “until a few years ago engineers were going to filing cabinets to get 30-year old data to start their analysis.”
Cotton said that instituting data governance procedures into an organization that is 33 years old is “the hardest part by far.” Some engineers, she said, have been working at the organization since the 1980s and “have some personal processes that they have not written down.”
“We are helping them institutionalize some of those ideas by putting it into code,” she said.
“Technology and management processes must go to together,” she said. “It’s important to identify technology that fits in the workflows, or they will not be adopted without a fight,” Cotton said.
She also said her organization needs to build a “future-proof solution … We can’t build a data lake architecture that is archaic in ten years, or even five.”
Central to the data management challenge is finding easier ways to share data more easily, Cotton said. “Finding the right file from ten years ago … is too hard,” she said. “The problem is crying out for some automation.”
With the benefit of automation helped by machine learning, “we are building a better data organization that allows us to bring messy unorganized legacy data to use to make decisions for the future,” she said. “It saves us labor…for us it’s not about profits, it’s about hours” that can be used to solve problems.
Asked how the organization is accepting the automation efforts, she said, “in the beginning it was very skeptical,” and that made it important to show some improvements early on in the process. Since then, however, the office’s engineers “became very excited about it … they need someone to help them.”
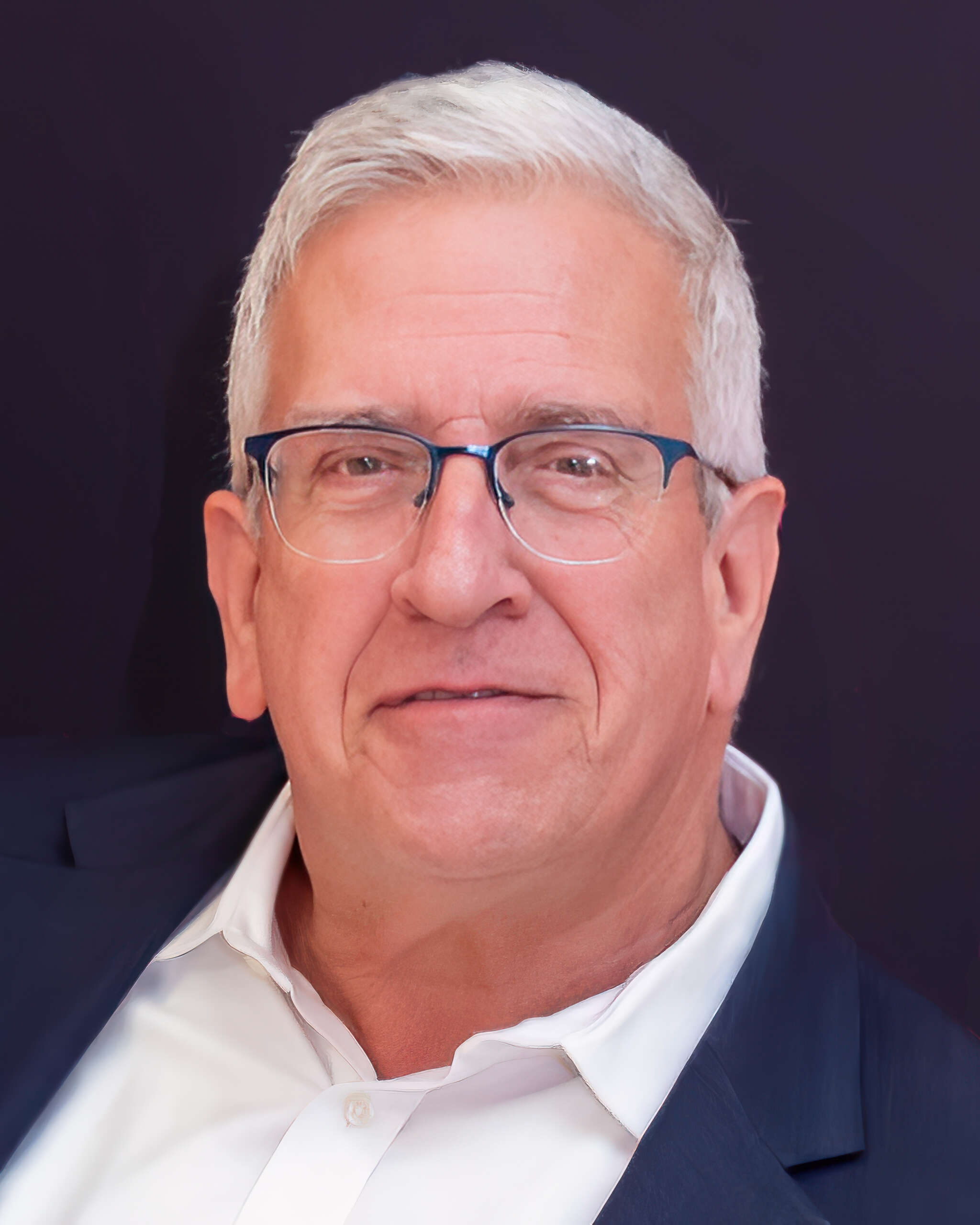