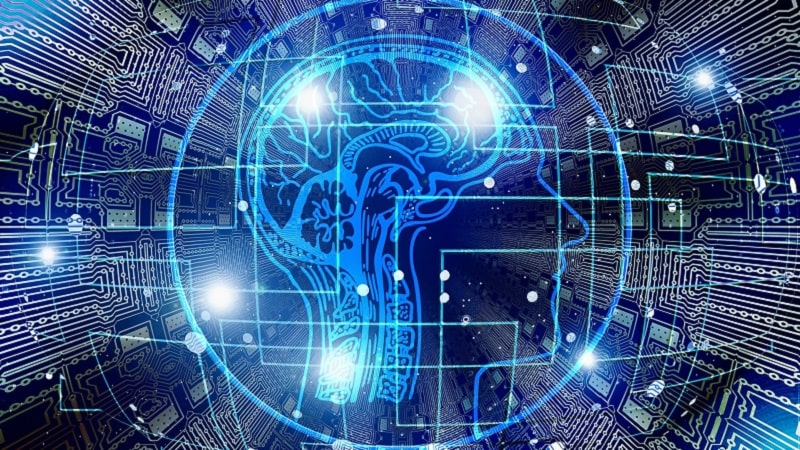
As advances in computing power and the ability to leverage large data sets and complex algorithms have increased in recent years, Federal agencies are embracing artificial intelligence (AI) to gain new insights from data and improve operational efficiencies in everything from healthcare to transportation to citizen services and public safety.
NASA used the technology to capture and process data for missions to Mars, using AI to develop improved systems and allow rovers to venture further and faster. The U.S. Patent and Trade Office is using AI tools to enhance the quality and efficiency of the patent and trademark examination process, while the National Oceanic and Atmospheric Administration is utilizing data-centric approaches to predict weather disasters and alert the public in real time.
The U.S. Nuclear Regulatory Commission is exploring ways AI can help detect cyberattacks on power plants. Storage solutions from DDN are also critical to a newly announced initiative with the Department of Energy, in which the Pacific Northwest National Laboratory will use AI to enhance the predictive understanding of coastal systems, including the response to short- and long-term changes.
Noted Rob Genkinger, vice president of program and strategy at DDN, “the promise of AI is that you get more accurate, more granular information to aid in decision-making. Another big promise is the ability to leverage massive amounts of data in real time. Whether it’s through automation or those better decisions, there’s real-world applicability for AI in agency mission delivery.”
Considering the Federal AI landscape, Pamela Isom, director of the AI and Technology Office at the Department of Energy, observed, “The Federal government as a whole, from my perspective, is making pretty good strides when it comes to understanding how to apply AI towards the mission.”
Architect for AI Success
AI represents “an intersection of new massive capabilities of storing data, incredibly fast transfer speeds across networks, and the vast amount of computing power we’re suddenly able to unleash on these datasets,” said Judson Graves, director of analytics and AI at ViON, during a recent panel discussion.
But getting from AI inspiration to AI project delivery can be a challenging process. Important questions need to be addressed up front, starting with where the AI project will live – on-premises or in the cloud.
When data sets and well-defined tools are already running in the cloud, leveraging the cloud for AI makes sense, Genkinger said. When the AI project relies on access to huge data sets from varying sources, the required data processing could overwhelm the cloud, with latency, performance, and data gravity all presenting challenges. In these instances, an on-premises deployment will end up yielding better results and being more cost effective, he advised.
“Even after you’ve considered those questions, the major thing that’s often overlooked is storage. Getting storage wrong will absolutely kill momentum on your AI development and require a tactical pause so you can identify your problems and determine a solution,” Genkinger said.
Often, to get a project started quickly, agencies look to leverage older on-premises infrastructure or existing cloud infrastructure. Just as often, the project achieves early success but suffers setbacks as the capability to process data quickly and manage capacity demands cannot keep up with the massive volumes of new data being generated.
Unlike traditional software programmed to perform linear tasks and deliver desired results based on specific instructions, AI is dynamic. It learns as it goes, and therefore requires concurrent processes in real time.
“With AI, you’ve got to do all sorts of things in parallel – pull data from storage, manipulate it, put it back, and then retest it, all while creating ever-expanding sets of new data and managing archives,” Genkinger said. Getting the architecture wrong compromises the AI initiative and frustrates data scientists, he observed.
Plan for Ballooning Data Volumes
Recently, Federal CIO Clare Martorana issued a call out to agencies requiring them to share how they are currently using AI as well as any projects that are on the horizon. The goal is to ensure that agencies are communicating to increase cross-agency learning and provide opportunities to leverage the data and the resulting discoveries as more projects are deployed.
To gain actionable insights, AI projects require robust data processing, as well as data governance that ensures data integrity, security, and availability – including the ability to share data and to house it in multitenant environments.
Data processing takes time and requires specialized skills. Data must be prepared, organized, and labeled in order to be trained and tested before an agency can derive insights from it. This requires people with the right skillsets to successfully develop, buy, or use AI capabilities. Data engineers, data scientists, storage engineers, and solution architects all play a role in achieving the desired outcomes from AI initiatives.
“It’s a continuous improvement process and requires a lot of volume and throughput, so the data must be running nonstop. Properly designed infrastructure will not only support these operational aspects, but also allow for collaboration, cooperation, and continuity,” Genkinger said.
The easiest way to avoid challenges when developing AI projects is to invest in time with experts, he advised. “You can talk to other agencies that had successful AI deployments and get best practices and ideas, so you’re not reinventing the wheel,” he said.