The Department of Energy’s (DoE) Office of Science, under the Nuclear Physics (NP) program, is looking to award about $22 million for research and development (R&D) efforts focused on AI and machine learning (ML) solutions for nuclear accelerators and detectors.
The agency said in its funding opportunity announcement that the AI/ML would enable the autonomous optimization and control of current and next-generation nuclear physics accelerator facilities and instruments. Additional AI/ML would help advance nuclear physics computations.
“Current and planned NP facilities and scientific instrumentation face a variety of technical challenges in theory, simulations, control, data acquisition, and data analysis,” said the DoE. “AI methods and techniques promise to address these challenges and shorten the timeline for experimental and computational discovery.”
The requested R&D would address challenges in autonomous control, accelerator and scientific instrument efficiency, and digital twinning for future colliders. It would also efficiently extract key insights from large data sets to drive new physics discoveries.
Funding recipients must submit periodic progress reports and present updates at an annual meeting during the two-year project, according to the document. Letters of intent are due on Nov. 14, 2024, and applications are due on Jan. 14, 2025.
DoE also highlighted its future interest in integrating “next-generation AI hardware and electronics” into detector systems.
The agency oversees four accelerator-based facilities, including the Relativistic Heavy Ion Collider at Brookhaven National Laboratory, the Continuous Electron Beam Accelerator Facility at the Thomas Jefferson National Accelerator Facility, the Argonne Tandem Linac Accelerator System at Argonne National Laboratory, and the Facility for Rare Isotope Beams Facility at Michigan State University.
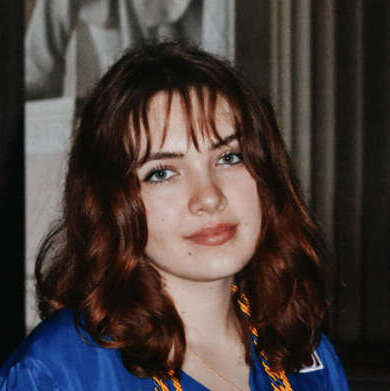