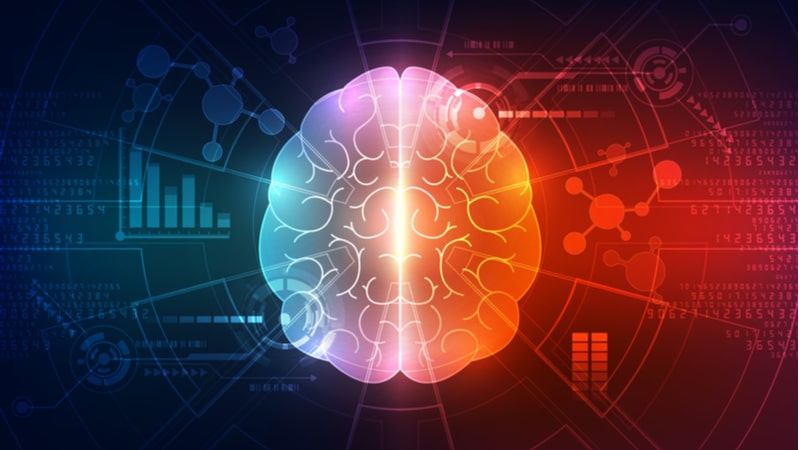
When NASA needed to land a probe on Mars, the landing sequence was the riskiest part of the multi-year, multi-billion-dollar mission-critical program. Prior to launch, NASA simulated the process under all potential weather and environmental conditions. Leaving nothing to chance, they used DDN storage solutions to fuel the artificial intelligence (AI) and advanced machine learning applications. After the successful landing on Mars, DDN was further entrusted with the data that made the 91-million-mile journey back to earth for analysis and other simulations.
Whether for ensuring the success of missions on Mars, investigating the impacts of coastal erosions, or other more terrestrial-based missions, DDN’s AI-optimized storage solution is at the heart of many critical Federal programs.
MeriTalk recently sat down with Rob Genkinger, vice president of program and strategy at DDN, for an in-depth discussion on the importance of mission-critical storage infrastructure decisions in successfully deploying and growing AI projects.
MeriTalk: What are the biggest drivers for AI in the Federal government today?
Rob Genkinger: Federal agencies are pushing to adopt AI technology for lots of different reasons.
Agencies are realizing they can achieve and enhance their missions by using a data-centric approach to tackle ever more complex problems, adopting AI techniques allows them to accelerate analysis and develop more accurate, data-driven insights to support strategic decision-making. With commercial organizations driving AI innovation, Federal agencies can and should leverage those technologies and bring strategic investment to ensure America’s AI leadership position.
Federal AI programs, supported by the recent bipartisan appropriations bill, which includes a further $1 billion in funding, will help fund investments in AI and machine learning. DDN is partnering with Federal agencies on defense, intelligence, energy, public health, and environmental programs to ensure that they can meet their data security and data management goals for the modernization of high-performance computing, physics research, and AI initiatives.
MeriTalk: What are the foundational elements of an AI-ready infrastructure?
Genkinger: The three foundational elements for a successful AI project include infrastructure (compute, networks, software, and storage), data, and people. You need all three, and they all need to be ready to do their job in order to have a successful AI project.
We are seeing the technologies and the processes maturing side by side. Early AI programs were built as monolithic, specialized systems, but increasingly, agencies need to build systems that can become more agile and evolve and expand to support more projects.
At DDN, we believe that a fully integrated AI platform, with full orchestration of compute, network, and storage, is the best way to support these evolving disciplines, to provide the tools for continuous delivery and optimization of AI services, and to allow users to innovate and focus on mission-critical outcomes.
MeriTalk: What should agencies that are starting to modernize legacy technology keep in mind as they work through their AI roadmap?
Genkinger: There are a few primary considerations. A common statistic is that nine out of 10 AI projects fail to reach production for one reason or another. We find that it is essential to set clear mission goals and objectives for AI programs.
We also find that data can overwhelm AI systems when they scale into production, so we recommend that agencies adopt a data-first strategy to plan for how they will collect, process, and archive that data at scale, ensuring AI programs can accelerate and achieve their objectives.
A common question is whether to host in the cloud or in an on-premises facility. Certain workloads will thrive in cloud-based systems. In other cases, the amount of data, and data throughput, may overwhelm the interconnect resources available in the cloud, and in those cases, an on-premises platform is going to be more effective in achieving mission goals. Performance and latency can be additional concerns when operating in cloud-based environments. Certain data-intensive workloads will demand an on-premises solution.
Agencies should draw on validated reference architectures to simplify integration and leverage experience and expertise from their technology partners. By collaborating and sharing experiences and best practices, agencies can build an AI Center of Excellence as a focus for expertise and resources.
By building an AI practice, agencies can learn and share with other experts who have seen projects from concept to architecture to execution and focus on data-engineering aspects of the mission to optimize data collection, processing, and governance across the end-to-end data lifecycle.
MeriTalk: What is the data lifecycle in an AI environment, and how does infrastructure affect the lifecycle?
Genkinger: The AI data lifecycle starts with data acquisition. There’s a ton of data out there, some usable, some not. It’s important to pick data that matters to give yourself a reasonable shot at solving your problem.
Next, you’re preparing the data. Once you’ve pulled the data, you’ve got to label it, clean it, and transform it. Then, you train it, score it, and then use the model to apply to live data to develop insights, recommendations and deliver outcomes.
It’s a continuous improvement process and requires a lot of volume and throughput, so the data must be running nonstop. The final step is storing the data for review, archive, and analysis. Properly designed infrastructure will not only support these operational aspects, but also allow for collaboration, cooperation, and continuity. With DDN, customers minimize data movement between each of these stages – which can be very costly in both time and money – by deploying a central data repository.
MeriTalk: What common missteps do you see in AI planning, and how can they be overcome?
Genkinger: We often see a pattern where an agency invests in a strategic AI program and starts using traditional IT infrastructure or the cloud to get started quickly. They skip the planning and design, hoping to see quick results.
Then, as their AI models grow, data management becomes more complex, and the system becomes slow and unmanageable. They try to add even more storage, but it’s not helping because the problem isn’t necessarily capacity. It’s the capacity, management, and governance of the data that has become untenable. It is harder to bring in new data, and the data scientists and analysts can’t get their job done.
The most important part of the project is the people. Data engineers, data scientists, storage engineers, and solution architects are all involved in driving the systems and data to deliver outcomes from an AI program. Few things are worse than having data scientists feel like they can’t do their job because of poor infrastructure that was simply designed for a quick start.
The easiest way to avoid these issues is to invest in time with experts. You can talk to other agencies that had successful AI deployments and get best practices and ideas, so you’re not reinventing the wheel.
MeriTalk: How do agencies balance the need to architect to solve a problem today and plan for future projects that they haven’t envisioned?
Genkinger: You can’t always predict the future, and not all existing storage solutions have the ability to support this type of growth. But with DDN, it’s easy to design an architecture to be consumable and composable, doing the small things first to explore the problem space, and then scale out as you gain confidence.
We have built a variety of solutions capable of addressing a wide range of requirements, ranging from individual systems to global-scale workloads. Many of our customers have systems that started with a couple hundred terabytes before needing to scale to petabytes, even hundreds of petabytes at full production. We encourage customers to start small, then scale later when they understand the size of the data required to deliver the outcomes they need.
MeriTalk: Let’s talk about DDN. What sets your company apart in terms of your approach to AI in the Federal government?
Genkinger: Trust. DDN is the trusted global leader in AI, with 20 years’ experience working with Federal agencies on high-performance analytics and big data management.
We’ve used that experience and invested in R&D to make data a lot easier to consume, manage, and monitor, so agencies can get started quickly with AI in a pilot program and then scale up and out dramatically – without needing specialist skills to run the infrastructure.
And with close collaborative partnerships with other AI technology providers, we bring together our seasoned experts with leading Federal systems integrators to build exascale systems for mission-critical AI.