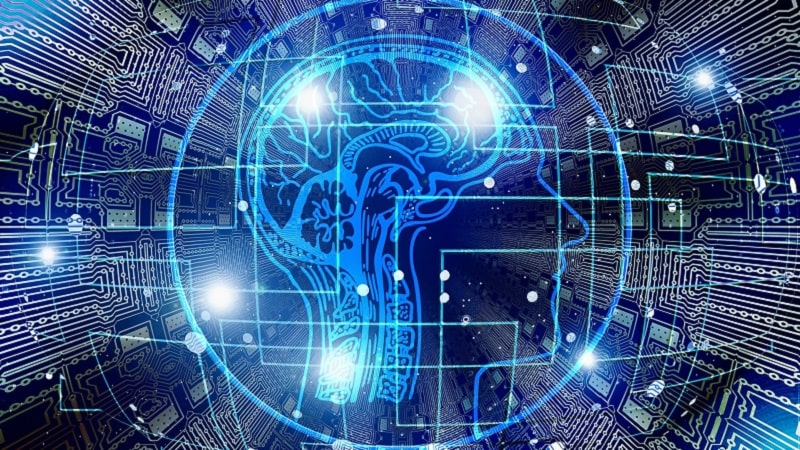
It is a truism in government that artificial intelligence (AI) and machine learning (ML) are the wave of the future, offering many opportunities for increased efficiency and accuracy that help to better serve the public. Early adopters in the Federal government have realized some quick wins with the technologies – but it is often a challenge to understand when those technologies are mature enough to be applied to a particular problem. MeriTalk sat down with James Bench, senior principal at Maximus, to assess Federal AI and ML adoption, how agencies can select the right projects to apply AI and ML, and how to build momentum around adoption and utilization.
MeriTalk: Can you talk a bit about some of the challenges that agencies are solving with AI and ML?
James Bench: One of the big win stories is the U.S. Postal Service. Using optical recognition and natural language processing, AI technology can look at handwritten envelopes and process 17.7 million pieces of mail an hour. The Securities and Exchange Commission (SEC) uses machine learning data science to identify and investigate fraud. It has a rich and large data set – filings from regulated entities along with external trading data, third-party databases, websites, and media reports. Fraud detection, especially credit fraud, has been a long-running capability for data science in general. U.S. Citizenship and Immigration Services (USCIS) has a virtual assistant chatbot supporting several languages and uses ML to enhance customer service and reduce Federal help desk labor. Leveraging longtime solutions of AI and ML in the commercial arena will continue to be a good fit for Federal agencies.
MeriTalk: And what are some of the biggest opportunities that these technologies offer?
James Bench: Currently, the unemployment rate is so low that it’s hard to find people for different tasks and positions. Agencies face a real lack of skillsets as they work to fulfill their missions. There are tremendous opportunities to use robotic process automation (RPA), chatbots, and automated functions to take on the routine, repetitive tasks and automate them. It doesn’t mean you’re taking jobs away; it means that you are leveraging these technologies to enable the current workforce to be more efficient and still serve the public.
If a person’s task has 10 steps and you can solve two with ML, you can reduce friction and make that task more efficient. If you do that with many different tasks, you start to save the workforce a lot of steps. The biggest opportunities are splitting up a multi-step process and automating a couple of steps.
MeriTalk: How can agencies address the need for re-skilling existing workers to better utilize AI and ML?
Bench: In general, the field is not mature enough to warrant agency development of a cohort of AI and ML data scientists. Agencies need to understand the capabilities of AI and ML and which areas are mature enough to be utilized to serve the mission. There are so many facets of the field. Some are emerging and sound promising, but they’re not pragmatic to implement. Others, like fraud detection, can be implemented right now. Understanding AI and ML can help the government identify where implementation would be beneficial.
In research, where the subject matter is new – like with the Defense Advanced Research Projects Agency (DARPA) programs – agencies do need AI and ML experts. But, in general, agencies just need to understand where the field is so they can avoid being sold an idea that becomes another pilot program or proof of concept that never gets implemented. We are seeing more of those now than ever before.
When agencies need the actual technical hard skills, that’s where using a system integrator such as Maximus makes sense because it’s harder for agencies to retain the talent. They can put the burden on the integrator to handle the actual implementation, the retention issues, and the cross-skilling.
MeriTalk: Are agencies currently organized in a way that can enable them to take full advantage of AI and ML? If not, what are some best practices to consider?
Bench: No, agencies are not ready to take full advantage of AI and ML. Implementation is still in the very early stages. In most cases, they are doing proof of concepts or trying to create a framework to better take advantage of the technology. To be successful with AI and ML, agencies need a new approach to project development. Typically, when Federal agencies receive appropriated funds, they also have a specific desired outcome.
When an IT team is doing AI and ML, it’s rarely that cut and dry. They will try something, and they don’t know if the data is good enough to support the conclusion that they’re trying to make. They’ll spend time to find out if the data is good enough and how close they can get to the answer they want. That learning and research mentality is a best practice. The process is trial and error, iterative, and agile. They may not get it right immediately. That’s part of the process; they’re going to learn from it and mature it. And if the data is not rich enough to help them draw the right conclusions, they want to find a way to get the data they need. If they can’t do that, then they know it’s time to try something else. This approach is hard to grasp unless you’re from a research environment where you try out different scenarios because it’s never been done before.
MeriTalk: Do you think understanding how AI and ML work is growing in the government?
Bench: Yes, this process is being talked about more. People will ask, “How did you get here?” And researchers will say, “It didn’t start easy. We didn’t knock it out of the park at first. It took a lot of learning to figure out how to get there.” If that discussion continues to happen, understanding will continue to grow, and the process will continue to evolve.
MeriTalk: How does Maximus help agencies get started with AI, ML, and RPA, and ensure a successful, seamless implementation?
Bench: In every project, we spend a lot of front-end time on education. For example, we might say, “Here’s where the data supports the use of AI, and it worked out this problem, which is similar to yours and has a high probability of success.” That education piece is important. It sets expectations about what is good enough to start with and what you want to mature into. Maximus supports that education.
We also encourage practical applications, those little wins to inspire new applications of AI and ML, and we want to inspire continuous innovation. As mentioned earlier, if a process involves 10 steps, a quick win means automation can do two of them. But continuous innovation asks, “What if I can do five?” You keep iterating, measuring, and marketing wins, and people start seeing that the technology can be used in far more places than it’s used today.
MeriTalk: How is Maximus’s approach to AI, ML, and RPA different from other companies?
Bench: When we’re working with clients on multibillion-dollar programs, we’re always looking for opportunities to use AI, ML, and RPA even though it may not have been in the project description. Maximus is different because we’re looking to implement AI and ML even in small pieces. We’re trying to implement it in nearly all our projects because we can leverage a lot of these capabilities and provide our customers with a strong use case.
For example, we have a contract at USCIS where we’re using ML for identity resolution. As non-citizens interact with the United States, they can do that in many different ways – through crossing borders, entering ports, trying to get a benefit or naturalization, or applying for a visa. USCIS needs to find all the interactions that person has had with the United States over the past 30 years.
To do that, we’re using ML, which takes on the repetitive task of hunting through systems to find all the encounters. The adjudicator doesn’t have to hunt the systems or figure out that James is Jim in this system and Jimmy in this other one. They don’t have to reconcile when a date of birth, SSN, or alien registration number was mistyped in one encounter. The ML does that work for you. It standardizes the hunting process. The adjudicator just looks at the whole timeline view of encounters and decides.
Our approach to leveraging these advanced technologies is focused on looking for those opportunities that begin the cycle: customer education, the quick wins, and the continuous innovation to help support the mission and help transform the future of government.