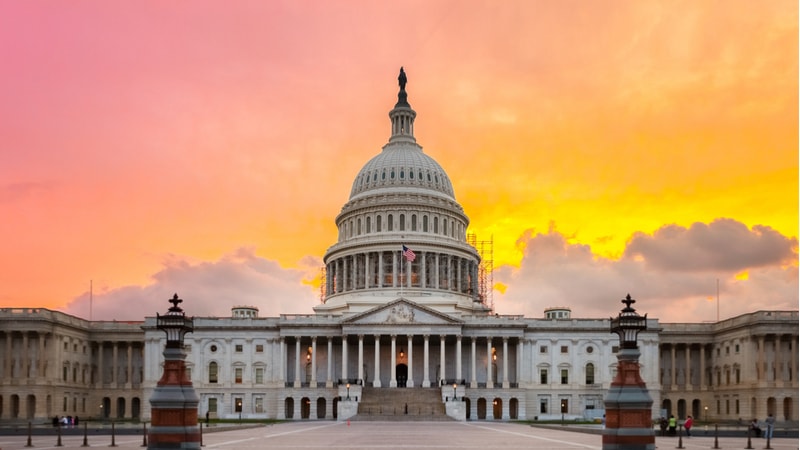
Artificial intelligence (AI) experts testified today before the House Financial Services Committee’s AI task force to help House members understand the risks associated with AI-based tools.
Chairman Rep. Bill Foster, D-Ill., had a particular interest in understanding the effects of slowing down AI implementations to examine the technology for possible risks thoroughly. He also asked witnesses of ways, if there are any, to preemptively reduce biases in predictive models, especially as organizations deal with the unintended negative consequences from AI-powered tools, such as algorithms that perpetuate baked-in racial or gender-based biases.
Meredith Broussard, an associate professor at New York University, recommended that companies take their time in evaluating AI tools rather than enthusiastically adopting every new technology in a mad scramble to emulate Big Tech firms.
However, according to Meg King, director of the Wilson Center’s science and technology innovation program, slowing the process is particularly difficult for private industries because it could cost them money. Enterprises cannot afford to slow down development to examine the nuances due to the current pace of advancement; a competitor might bring a similar product to market faster.
Therefore, to mitigate the harms of AI, King recommends that businesses and agencies implement an AI ethical framework. While the Department of Defense and other Federal agencies have worked towards putting together Ethical AI guidelines and frameworks, to date, there is no significant incentive for the private sector to include ethics directly in the AI development process.
Growing concerns about the technology have led some private companies to develop and deploy their own ethical frameworks for AI. But according to King, many of these frameworks are incredibly vague and include very little guidance as to application.
“No ethical AI framework should be static; as AI systems will continue to evolve, as will our interaction with them. Key components, however, should be consistent,” King said. That list, specifically for the financial sector, should include: explainability, data inputs, testing, and system lifecycle, she added.
Jeffery Yong, principal advisor at the Financial Stability Institute in the Bank for International Settlements, reinforced King’s statement. He added that there is a possibility to define financial regulatory expectations related to fairness and ethics.
“These could supplement consumer protection laws that cover non-discrimination clauses, which could also apply in the context of the use of AI in the financial sector,” he said. However, the level of complexity and lack of explainability that characterize AI models, Yong added, poses a challenge.
“A way to overcome these challenges is to consider a tailored and coordinated regulatory and supervisory approach. This means differentiating the regulatory and supervisory treatment on the use of AI models, depending on the conduct and prudential risks that they pose,” Yong said.
But AI systems do not all pose an equal risk of harm. Therefore, each system should be thoroughly and independently evaluated based on the level of risk to consumers, King added.
In addition, Broussard backed an approach to classifying AI applications into high- and low-risk categories; the EU proposed a similar regulation.
A low-risk use of facial recognition might be to unlock a phone, which has a backup method in case something goes wrong. High-risk usage of facial recognition might be law enforcement using facial recognition on real-time surveillance video feeds. Facial recognition technology has misidentified people with darker skin, showing that people of color are at a high risk of being harmed by facial recognition when used in policing. High-risk AI would need to be registered and regularly audited to ensure it is not harming citizens.
“I would recommend the U.S. adopts a similar strategy of characterizing AI as the high-risk and low-risk and regulating the high-risk uses in each industry. [However], after deciding which AI gets regulated, it is necessary to look for specific kinds of bias,” Broussard said.